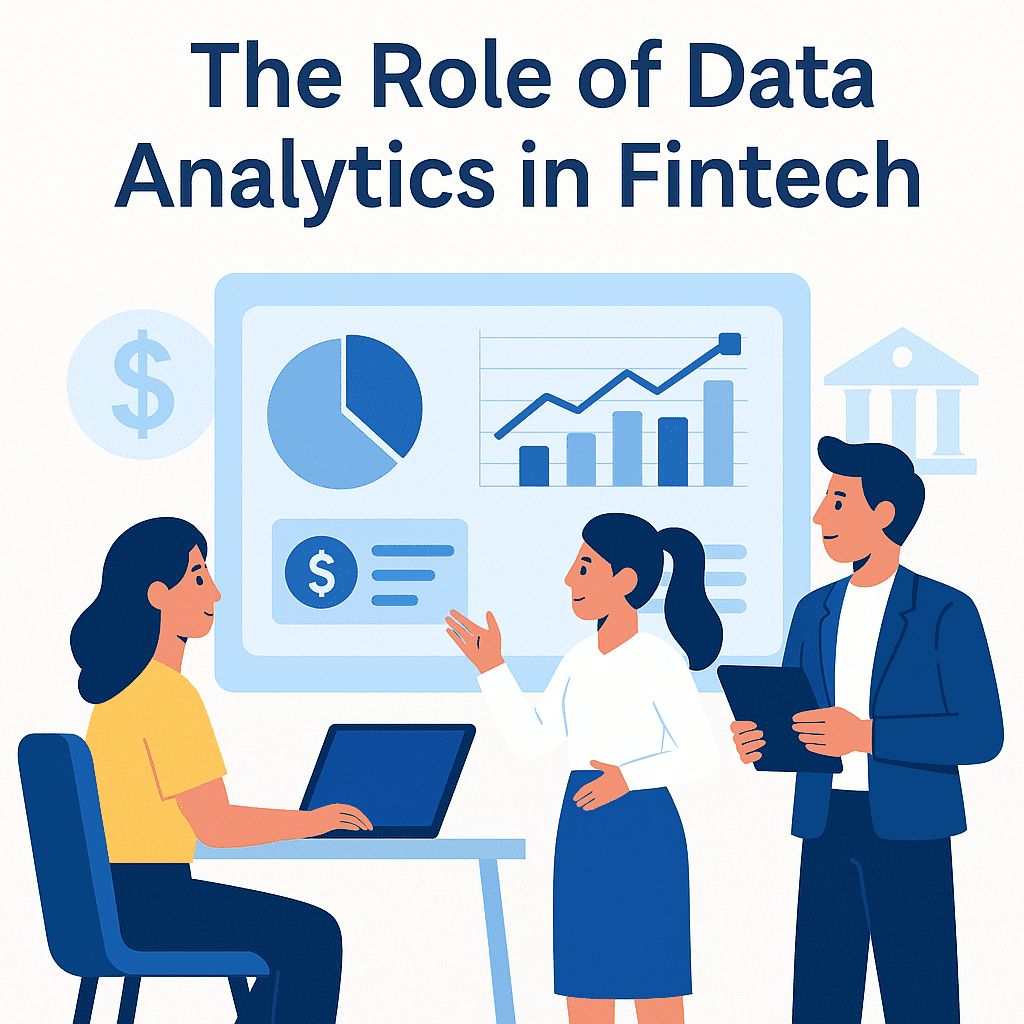
Introduction
In recent years, data analytics has emerged as a powerful force shaping the financial technology (fintech) industry. From personalized customer experiences to enhanced risk management and operational efficiency, data analytics has revolutionized how fintech companies operate. But what exactly is data analytics, and why is it so important in fintech?
Definition of Data Analytics and Its Relevance to the Fintech Industry
Data analytics refers to the process of examining large sets of data to uncover hidden patterns, trends, and insights. In the fintech industry, data analytics plays a crucial role in helping companies make informed decisions, improve customer experiences, and drive innovation. By analyzing data from various sources, such as customer transactions, social media interactions, and market trends, fintech companies can gain valuable insights that enable them to create more personalized, efficient, and secure financial services.
Latin America is an excellent example of a region where data analytics is significantly impacting fintech. With a rapidly growing digital economy, fintech companies in Latin America are leveraging data analytics to address unique challenges, such as financial inclusion, regulatory compliance, and customer satisfaction.
Importance of Data-Driven Decision-Making in Fintech
In the fintech industry, data-driven decision-making is vital for success. By utilizing data analytics, companies can make informed choices that lead to better customer experiences, increased efficiency, and reduced risks. This approach helps fintech companies to:
- Identify Customer Needs: By analyzing customer data, fintech companies can identify trends and preferences, allowing them to create personalized products and services that meet individual needs.
- Improve Operational Efficiency: Data analytics helps fintech companies optimize processes and automate tasks, reducing costs and improving efficiency.
- Manage Risks: Through predictive analytics, fintech companies can assess potential risks and implement strategies to mitigate them, ensuring the security and stability of their operations.
- Enhance Customer Experience: By leveraging data insights, fintech companies can create tailored experiences that cater to the specific needs and preferences of their customers.
In this article, we’ll explore how data analytics is transforming fintech in Latin America, focusing on real-world examples and applications that illustrate its impact.
Enhancing Customer Experience
One of the most significant ways data analytics is revolutionizing fintech in Latin America is by enhancing customer experiences. By analyzing customer data, fintech companies can offer personalized financial services and products that meet the unique needs of their customers.
How Data Analytics Enables Fintech Companies to Personalize Financial Services and Products
Personalization is a key driver of customer satisfaction in the fintech industry. Data analytics allows companies to analyze vast amounts of customer data, including spending habits, preferences, and behaviors, to create personalized financial services and products.
For example, Banco Itaú, a leading bank in Brazil, uses data analytics to offer personalized financial solutions to its customers. By analyzing transaction data, Banco Itaú can provide tailored product recommendations and financial advice, helping customers make informed financial decisions. This approach enhances customer satisfaction and fosters long-term relationships.
Examples of Personalized Recommendations and Tailored Financial Advice
Several fintech companies in Latin America are using data analytics to provide personalized recommendations and tailored financial advice:
- NuBank: NuBank, a digital bank based in Brazil, leverages data analytics to offer personalized financial products and services. By analyzing customer spending patterns, NuBank provides tailored recommendations for credit cards, loans, and investment opportunities. This personalized approach has helped NuBank become one of the leading fintech companies in Latin America, with a strong customer base and high satisfaction rates. You can learn more about NuBank’s personalized offerings here.
- Kubo.financiero: Kubo.financiero, a Mexican fintech company, uses data analytics to offer personalized investment advice. By analyzing customer risk profiles and financial goals, Kubo.financiero provides tailored investment recommendations that align with individual preferences and objectives. This personalized approach has made Kubo.financiero a popular choice among investors seeking customized financial solutions.
- Ualá: Ualá, an Argentine fintech company, uses data analytics to offer personalized financial advice and spending insights. By analyzing transaction data, Ualá provides customers with detailed spending reports and personalized recommendations to help them manage their finances effectively. This data-driven approach has contributed to Ualá’s rapid growth and popularity in Argentina.
Use of Data Analytics to Segment Customers Based on Their Behavior, Preferences, and Needs
Customer segmentation is a crucial aspect of personalization in fintech. Data analytics enables companies to segment customers based on their behavior, preferences, and needs, allowing for more targeted marketing and customized user experiences.
For instance, Creditas, a Brazilian fintech company specializing in secured loans, uses data analytics to segment its customers based on factors such as creditworthiness, income, and financial goals. By understanding the unique characteristics of each segment, Creditas can offer tailored loan products and personalized financial advice that cater to the specific needs of its customers. This targeted approach has helped Creditas become a leading player in the Brazilian lending market.
Benefits of Targeted Marketing and Customized User Experiences
Targeted marketing and customized user experiences offer numerous benefits to fintech companies and their customers:
- Increased Customer Satisfaction: By providing personalized products and services, fintech companies can enhance customer satisfaction and build long-term relationships.
- Higher Conversion Rates: Targeted marketing campaigns are more likely to resonate with customers, leading to higher conversion rates and increased sales.
- Improved Customer Retention: Customized user experiences foster customer loyalty and retention by meeting individual needs and preferences.
- Enhanced Brand Loyalty: Personalized experiences create a strong emotional connection with customers, leading to increased brand loyalty and advocacy.
A great example of this is Mercado Libre, the largest e-commerce and fintech company in Latin America. Mercado Libre uses data analytics to segment its users based on their purchasing behaviour and preferences, allowing for targeted marketing campaigns and personalized product recommendations. This approach has contributed to Mercado Libre’s success in attracting and retaining millions of customers across the region.
Risk Management and Fraud Detection
In the fintech industry, risk management and fraud detection are critical to ensuring the security and stability of financial operations. Data analytics plays a vital role in helping fintech companies identify and mitigate risks, detect fraudulent activities, and protect customer information.
Application of Predictive Analytics to Assess and Manage Financial Risks
Predictive analytics involves using historical data and statistical algorithms to predict future outcomes. In fintech, predictive analytics is used to assess and manage financial risks, enabling companies to make informed decisions and implement strategies to mitigate potential threats.
Kueski, a Mexican online lending platform, leverages predictive analytics to assess credit risks and determine loan eligibility. By analyzing data on customer behavior, financial history, and social media activity, Kueski can accurately predict the likelihood of loan repayment and assess the risk of default. This data-driven approach allows Kueski to offer loans to underserved segments while minimizing risk exposure. You can learn more about Kueski’s predictive analytics capabilities here.
Techniques for Identifying Potential Default Risks and Fraudulent Activities
Data analytics provides fintech companies with powerful tools and techniques to identify potential default risks and fraudulent activities:
- Machine Learning Algorithms: Machine learning algorithms can analyze vast amounts of data to identify patterns and anomalies, enabling fintech companies to detect potential risks and fraud. For example, Konfio, a Mexican fintech company specializing in small business loans, uses machine learning algorithms to assess credit risks and detect fraudulent activities. By analyzing data on customer behavior and financial transactions, Konfio can identify potential risks and take proactive measures to mitigate them.
- Network Analysis: Network analysis involves examining relationships between data points to identify suspicious patterns and connections. In the fintech industry, network analysis is used to detect fraudulent activities by analyzing transaction networks and identifying unusual patterns.
- Behavioral Analytics: Behavioral analytics focuses on analyzing customer behavior to identify anomalies and potential risks. By understanding typical customer behavior, fintech companies can detect unusual activities that may indicate fraud or security threats.
Implementation of Real-Time Data Analytics to Monitor Transactions and Detect Anomalies
Real-time data analytics is essential for monitoring transactions and detecting anomalies in the fintech industry. By analyzing data as it arrives, fintech companies can identify suspicious activities and respond promptly to mitigate risks.
CrediJusto, a Mexican fintech company offering loans to small businesses, uses real-time data analytics to monitor transactions and detect anomalies. By analyzing data on financial transactions, customer behavior, and market trends, CrediJusto can identify potential risks and fraudulent activities, ensuring the security and stability of its operations.
Examples of Fraud Detection Systems and Their Effectiveness
Several fintech companies in Latin America have implemented effective fraud detection systems using data analytics:
- Banco do Brasil: Banco do Brasil, one of the largest banks in Brazil, uses data analytics to detect fraudulent activities and protect customer information. By analyzing transaction data and customer behavior, Banco do Brasil can identify suspicious activities and take immediate action to prevent fraud. This data-driven approach has significantly reduced fraud rates and enhanced customer trust. Learn more about Banco do Brasil’s fraud detection systems here.
- Clip: Clip, a Mexican fintech company specializing in payment solutions, uses data analytics to detect and prevent fraudulent transactions. By analyzing transaction data and identifying patterns, Clip can detect anomalies and take proactive measures to protect customers and merchants from fraud. This approach has made Clip a trusted payment solution provider in Mexico. You can read more about Clip’s fraud detection capabilities here.
- Mercado Pago: Mercado Pago, the fintech arm of Mercado Libre, uses data analytics to detect and prevent fraudulent activities in its payment platform. By analyzing transaction data and customer behavior, Mercado Pago can identify suspicious activities and implement security measures to protect users. This data-driven approach has helped Mercado Pago become a leading payment platform in Latin America. Discover more about Mercado Pago’s fraud prevention strategies here.
Optimizing Operations and Efficiency
Data analytics plays a crucial role in optimizing operations and enhancing efficiency in the fintech industry. By leveraging data insights, fintech companies can streamline processes, automate workflows, and improve overall operational performance.
How Data Analytics Drives Process Automation and Operational Efficiency
Process automation is a key driver of operational efficiency in fintech. By automating repetitive tasks and processes, fintech companies can reduce costs, improve accuracy, and enhance overall efficiency.
EBANX, a Brazilian fintech company specializing in cross-border payment solutions, uses data analytics to drive process automation and optimize operations. By analyzing data on customer transactions, payment methods, and market trends, EBANX can identify opportunities for automation and implement efficient workflows. This data-driven approach has enabled EBANX to offer seamless payment experiences to customers across Latin America. Learn more about EBANX’s process automation strategies here.
Use Cases of Automated Workflows and Decision-Making Processes
Data analytics enables fintech companies to implement automated workflows and decision-making processes that enhance operational efficiency:
- Loan Processing: Fintech companies like Creditas in Brazil use data analytics to automate loan processing workflows. By analyzing customer data and credit histories, Creditas can automate loan approval processes, reducing processing time and improving efficiency. This automated approach allows Creditas to offer quick and seamless loan services to its customers.
- Customer Support: Fintech companies like Ualá in Argentina use data analytics to automate customer support processes. By analyzing customer inquiries and interactions, Ualá can implement chatbots and automated responses that address common customer issues, improving response times and customer satisfaction.
- Fraud Detection: Fintech companies like Konfio in Mexico use data analytics to automate fraud detection processes. By analyzing transaction data and identifying suspicious patterns, Konfio can implement automated fraud detection systems that flag potential risks and prevent fraudulent activities. This automated approach enhances security and reduces manual intervention.
Leveraging Data Analytics to Track and Analyze Key Performance Indicators (KPIs)
Key performance indicators (KPIs) are essential metrics that help fintech companies measure and evaluate their performance. Data analytics enables companies to track and analyze KPIs, providing valuable insights into business operations and performance.
Nubank, a Brazilian digital bank, leverages data analytics to track and analyze KPIs such as customer acquisition, retention rates, and transaction volumes. By monitoring these metrics, Nubank can identify areas for improvement and implement strategies to enhance performance and customer satisfaction. This data-driven approach has contributed to Nubank’s rapid growth and success in the Latin American market.
Continuous Improvement Through Data-Driven Insights and Feedback Loops
Continuous improvement is a key aspect of operational efficiency in fintech. Data analytics enables companies to gather insights and feedback, facilitating continuous improvement and innovation.
Kubo.financiero, a Mexican fintech company, uses data analytics to gather customer feedback and insights. By analyzing customer interactions and feedback, Kubo.financiero can identify areas for improvement and implement changes to enhance customer experiences. This data-driven approach has helped Kubo.financiero maintain high levels of customer satisfaction and loyalty.
Enhancing Financial Products and Services
Data analytics plays a vital role in enhancing financial products and services in the fintech industry. By leveraging data insights, fintech companies can develop innovative products, gather customer feedback, and improve overall product offerings.
Role of Data Analytics in Developing and Refining Financial Products and Services
Data analytics enables fintech companies to develop and refine financial products and services that meet the evolving needs of customers. By analyzing customer data and market trends, companies can identify opportunities for innovation and create products that offer value to customers.
Mercado Pago, the fintech arm of Mercado Libre, uses data analytics to develop and refine its financial products and services. By analyzing transaction data and customer preferences, Mercado Pago can identify new product opportunities and enhance existing offerings. This data-driven approach has contributed to Mercado Pago’s success as a leading payment platform in Latin America.
Case Studies of Successful Product Innovations Driven by Data Insights
Several fintech companies in Latin America have successfully leveraged data analytics to drive product innovations:
- EBANX: EBANX, a Brazilian fintech company, used data analytics to develop its cross-border payment solutions. By analyzing data on customer transactions and payment methods, EBANX identified the need for seamless payment experiences for international customers. This insight led to the development of innovative payment solutions that cater to the unique needs of Latin American businesses and consumers. You can read more about EBANX’s product innovations here.
- Kueski: Kueski, a Mexican online lending platform, used data analytics to develop its instant loan products. By analyzing customer data and financial histories, Kueski identified the demand for quick and accessible loans, leading to the creation of instant loan products that cater to underserved segments. This data-driven approach has made Kueski a popular choice for online lending in Mexico. Learn more about Kueski’s product innovations here.
- NuBank: NuBank, a Brazilian digital bank, used data analytics to develop its credit card offerings. By analyzing customer spending patterns and preferences, NuBank identified the need for flexible and transparent credit card solutions, leading to the creation of products that offer no annual fees and real-time spending insights. This data-driven approach has contributed to NuBank’s rapid growth and popularity in Brazil. Discover more about NuBank’s product innovations here.
Using Data Analytics to Gather and Analyze Customer Feedback for Product Improvement
Customer feedback is essential for product improvement and innovation in fintech. Data analytics enables companies to gather and analyze feedback, providing valuable insights into customer preferences and areas for improvement.
Ualá, an Argentine fintech company, uses data analytics to gather customer feedback and insights. By analyzing customer interactions and feedback, Ualá can identify areas for improvement and implement changes to enhance its financial products and services. This data-driven approach has helped Ualá maintain high levels of customer satisfaction and loyalty.
Techniques for Sentiment Analysis and Customer Satisfaction Measurement
Sentiment analysis and customer satisfaction measurement are essential techniques for understanding customer preferences and improving products in the fintech industry:
- Sentiment Analysis: Sentiment analysis involves analyzing customer feedback and social media interactions to understand customer sentiments and opinions. Fintech companies can use sentiment analysis to identify customer preferences and areas for improvement.
- Net Promoter Score (NPS): NPS is a popular metric used to measure customer satisfaction and loyalty. By analyzing NPS data, fintech companies can identify areas for improvement and implement strategies to enhance customer experiences.
- Customer Surveys: Customer surveys are an effective way to gather feedback and insights. By analyzing survey data, fintech companies can identify customer preferences and areas for product improvement.
Creditas, a Brazilian fintech company, uses sentiment analysis and customer surveys to gather feedback and improve its financial products and services. By analyzing customer sentiments and preferences, Creditas can implement changes that enhance customer satisfaction and loyalty.
Compliance and Regulatory Reporting
Compliance with financial regulations and effective regulatory reporting are critical aspects of the fintech industry. Data analytics plays a vital role in ensuring compliance, maintaining data integrity, and facilitating regulatory reporting.
Importance of Data Analytics in Ensuring Compliance with Financial Regulations
Financial regulations are essential for maintaining trust and stability in the fintech industry. Data analytics enables fintech companies to ensure compliance with regulations and maintain transparency in their operations.
Banco Santander Mexico, a leading bank in Mexico, uses data analytics to ensure compliance with financial regulations. By analyzing transaction data and customer behavior, Banco Santander can identify potential compliance issues and implement measures to address them. This data-driven approach ensures compliance and enhances customer trust. Learn more about Banco Santander’s compliance strategies here.
Tools and Techniques for Regulatory Reporting and Audit Trails
Data analytics provides fintech companies with powerful tools and techniques for regulatory reporting and maintaining audit trails:
- Data Aggregation: Data aggregation involves collecting and consolidating data from various sources for regulatory reporting. Fintech companies can use data aggregation tools to streamline reporting processes and ensure accuracy.
- Audit Trails: Audit trails are records of transactions and activities that provide transparency and accountability. Data analytics enables fintech companies to maintain audit trails that facilitate regulatory compliance and reporting.
Mercado Pago, the fintech arm of Mercado Libre, uses data analytics to maintain audit trails and facilitate regulatory reporting. By analyzing transaction data and maintaining detailed records, Mercado Pago ensures compliance with financial regulations and enhances transparency.
Best Practices for Data Governance and Maintaining Data Integrity
Data governance and maintaining data integrity are critical aspects of compliance in the fintech industry. Data analytics enables companies to implement best practices that ensure data accuracy, security, and compliance:
- Data Quality Assurance: Fintech companies should implement data quality assurance processes to ensure the accuracy and reliability of data. This involves regular data validation, cleansing, and monitoring.
- Data Security: Data security is essential for protecting customer information and maintaining compliance. Fintech companies should implement robust security measures to protect data from unauthorized access and breaches.
- Data Privacy: Data privacy is a critical aspect of compliance in the fintech industry. Companies should implement privacy policies and practices that protect customer information and comply with data protection regulations.
Nubank, a Brazilian digital bank, follows best practices for data governance and maintaining data integrity. By implementing data quality assurance processes and robust security measures, Nubank ensures compliance with regulations and protects customer information. Learn more about Nubank’s data governance practices here.
Role of Data Analytics in Data Privacy and Security Compliance
Data privacy and security compliance are essential for maintaining customer trust and protecting sensitive information in the fintech industry. Data analytics plays a vital role in ensuring compliance and safeguarding customer data.
Banco Itaú, a leading bank in Brazil, uses data analytics to ensure data privacy and security compliance. By analyzing data on customer interactions and transactions, Banco Itaú can identify potential security threats and implement measures to protect customer information. This data-driven approach ensures compliance and enhances customer trust.
Investment and Wealth Management
Data analytics has transformed investment and wealth management in the fintech industry. By leveraging data insights, fintech companies can optimize investment portfolios, assess risks, and offer personalized financial advice.
Application of Data Analytics in Optimizing Investment Portfolios and Asset Allocation
Data analytics enables fintech companies to optimize investment portfolios and asset allocation by analyzing market trends, customer preferences, and risk profiles.
Ualá, an Argentine fintech company, uses data analytics to optimize investment portfolios for its customers. By analyzing data on market trends and customer risk profiles, Ualá can offer personalized investment recommendations that align with individual preferences and goals. This data-driven approach enhances investment outcomes and customer satisfaction.
Examples of Robo-Advisors and Algorithmic Trading Platforms
Robo-advisors and algorithmic trading platforms are popular fintech innovations that leverage data analytics to offer automated investment solutions:
- NuInvest: NuInvest, the investment arm of NuBank in Brazil, uses data analytics to offer robo-advisory services that provide personalized investment advice and portfolio management. By analyzing customer risk profiles and financial goals, NuInvest offers tailored investment solutions that cater to individual needs. Discover more about NuInvest’s robo-advisory services here.
- Kubo.financiero: Kubo.financiero, a Mexican fintech company, uses data analytics to offer algorithmic trading platforms that provide automated investment strategies. By analyzing market trends and customer preferences, Kubo.financiero offers investment solutions that optimize returns and minimize risks.
Use of Data Analytics to Assess and Mitigate Investment Risks
Data analytics enables fintech companies to assess and mitigate investment risks by analyzing market trends, customer risk profiles, and historical data.
Creditas, a Brazilian fintech company, uses data analytics to assess and mitigate investment risks for its customers. By analyzing data on market trends and customer preferences, Creditas offers personalized investment solutions that align with individual risk profiles and goals. This data-driven approach enhances investment outcomes and reduces risks.
Techniques for Stress Testing and Scenario Analysis
Stress testing and scenario analysis are essential techniques for assessing investment risks and ensuring portfolio resilience in the fintech industry:
- Stress Testing: Stress testing involves simulating adverse market conditions to assess the resilience of investment portfolios. Fintech companies use data analytics to conduct stress tests and identify potential vulnerabilities in portfolios.
- Scenario Analysis: Scenario analysis involves evaluating potential market scenarios and their impact on investment portfolios. By analyzing data on market trends and customer preferences, fintech companies can conduct scenario analyses that inform investment strategies.
Mercado Pago, the fintech arm of Mercado Libre, uses stress testing and scenario analysis to assess investment risks and optimize portfolios for its customers. By analyzing market trends and conducting scenario analyses, Mercado Pago offers personalized investment solutions that align with individual risk profiles and goals.
Challenges and Considerations
While data analytics offers numerous benefits to the fintech industry, there are also challenges and considerations that companies must address to ensure effective implementation and compliance.
Importance of High-Quality, Accurate Data for Effective Analytics
High-quality, accurate data is essential for effective data analytics in fintech. Without reliable data, companies cannot gain meaningful insights or make informed decisions.
Konfio, a Mexican fintech company, emphasizes the importance of data quality and accuracy in its operations. By implementing data validation and cleansing processes, Konfio ensures the reliability of its data, enabling effective analytics and decision-making.
Strategies for Ensuring Data Quality and Addressing Data Silos
Data quality and data silos are critical challenges in the fintech industry. Companies must implement strategies to ensure data quality and address data silos that hinder effective analytics:
- Data Integration: Data integration involves consolidating data from various sources to create a unified view. Fintech companies can use data integration tools to address data silos and ensure data accuracy.
- Data Validation: Data validation involves verifying the accuracy and completeness of data. Fintech companies should implement data validation processes to ensure data quality and reliability.
- Data Cleansing: Data cleansing involves removing duplicate or inaccurate data from datasets. Fintech companies should implement data cleansing processes to ensure data quality and accuracy.
Banco Santander Mexico, a leading bank in Mexico, follows best practices for data integration and validation to ensure data quality and address data silos. By implementing data governance processes, Banco Santander ensures the reliability and accuracy of its data. Learn more about Banco Santander’s data quality strategies here.
Balancing Data Analytics with Customer Privacy and Data Security
Balancing data analytics with customer privacy and data security is essential for maintaining trust and compliance in the fintech industry. Companies must implement robust security measures to protect customer information while leveraging data insights.
Banco Itaú, a leading bank in Brazil, prioritizes data privacy and security in its operations. By implementing robust security measures and privacy policies, Banco Itaú ensures compliance with regulations and protects customer information.
Compliance with Data Protection Regulations and Ethical Considerations
Compliance with data protection regulations and ethical considerations is critical for maintaining trust and transparency in the fintech industry. Companies must adhere to data protection regulations and ethical standards to ensure compliance and protect customer information.
Nubank, a Brazilian digital bank, follows best practices for compliance with data protection regulations and ethical considerations. By implementing privacy policies and ethical guidelines, Nubank ensures compliance and protects customer information.
Future Trends in Data Analytics for Fintech
The future of data analytics in fintech is promising, with numerous trends and advancements shaping the industry’s landscape. By staying abreast of these trends, fintech companies can leverage data analytics for innovation and growth.
Future Trends in Data Analytics for Fintech
Several future trends in data analytics are poised to shape the fintech industry:
- Artificial Intelligence and Machine Learning: Artificial intelligence (AI) and machine learning (ML) are set to revolutionize data analytics in fintech. By leveraging AI and ML algorithms, fintech companies can gain deeper insights, automate processes, and enhance decision-making.
- Real-Time Data Processing: Real-time data processing is becoming increasingly important in fintech. By analyzing data as it arrives, companies can identify trends, detect anomalies, and respond promptly to market changes. Learn more about real-time data processing trends in fintech here.
- Big Data and Advanced Analytics: Big data and advanced analytics are driving innovation in fintech. By harnessing large volumes of data and applying advanced analytics techniques, companies can gain actionable insights and optimize their operations. Discover more about big data trends in fintech here.
- Blockchain and Distributed Ledger Technology: Blockchain and distributed ledger technology (DLT) are gaining traction in fintech. By leveraging blockchain technology, companies can enhance transparency, security, and efficiency in financial transactions. Learn more about blockchain trends in fintech here.
Opportunities for Fintech Companies to Leverage Data Analytics
Data analytics presents numerous opportunities for fintech companies to innovate and grow:
- Personalization and Customer Experience: By leveraging data insights, fintech companies can offer personalized products and services that cater to individual customer preferences. This enhances customer experiences and drives loyalty.
- Risk Management and Fraud Prevention: Data analytics enables fintech companies to identify and mitigate risks, enhancing security and reducing fraud. By analyzing transaction data and customer behaviour, companies can implement effective risk management strategies.
- Operational Efficiency and Cost Reduction: Data analytics allows fintech companies to optimize operations, automate processes, and reduce costs. By streamlining workflows and enhancing efficiency, companies can improve their bottom line.
- Product Innovation and Development: Data analytics enables fintech companies to develop innovative products and services that meet customer needs. By analyzing market trends and customer preferences, companies can identify opportunities for innovation and create value-added solutions.
Potential Challenges and Solutions in Implementing Data Analytics
While data analytics offers numerous benefits, there are also challenges that fintech companies must address:
- Data Quality and Integration: Ensuring data quality and integration can be challenging for fintech companies. Implementing robust data governance processes and leveraging data integration tools can address these challenges.
- Privacy and Security Concerns: Balancing data analytics with customer privacy and security is critical. Fintech companies should implement robust security measures and privacy policies to protect customer information.
- Compliance with Regulations: Compliance with data protection regulations and ethical considerations is essential. Fintech companies must adhere to regulations and ethical standards to ensure compliance and maintain trust.
- Skill Gaps and Talent Acquisition: The demand for skilled data analysts and data scientists is increasing in the fintech industry. Companies should invest in talent acquisition and training to bridge skill gaps and leverage data analytics effectively.
Emerging Technologies Shaping the Future of Fintech
Several emerging technologies are poised to shape the future of fintech:
- Artificial Intelligence and Machine Learning: AI and ML are transforming data analytics in fintech. By leveraging AI and ML algorithms, companies can gain deeper insights, automate processes, and enhance decision-making.
- Blockchain and Distributed Ledger Technology: Blockchain and DLT are revolutionizing financial transactions. By enhancing transparency, security, and efficiency, blockchain technology is reshaping the fintech landscape.
- Internet of Things (IoT): IoT is creating new opportunities for data collection and analysis in fintech. By leveraging IoT devices, companies can gather real-time data and gain actionable insights.
- Quantum Computing: Quantum computing has the potential to revolutionize data analytics in fintech. By offering unprecedented computational power, quantum computing can solve complex problems and drive innovation.
Conclusion
Data analytics is transforming the fintech industry, offering numerous benefits and opportunities for innovation and growth. By leveraging data insights, fintech companies can enhance customer experiences, optimize operations, and develop innovative products and services. However, companies must also address challenges related to data quality, privacy, and compliance to ensure effective implementation and compliance.
Key Takeaways
- Understanding the Role of Data Analytics in Fintech: Data analytics is a powerful tool for understanding customer behavior, optimizing operations, and enhancing financial products and services.
- Benefits of Data-Driven Insights: Data-driven insights enable fintech companies to offer personalized experiences, optimize operations, and drive innovation.
- Challenges and Considerations: Ensuring data quality, privacy, and compliance is critical for effective data analytics implementation.
- Future Trends and Opportunities: Emerging technologies such as AI, blockchain, and IoT are shaping the future of fintech, offering new opportunities for innovation and growth.